Choice of unmanned aerial vehicles for identification of mosquito breeding sites
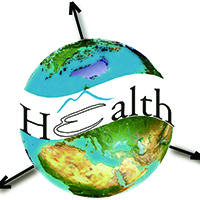
Submitted: 27 August 2019
Accepted: 12 April 2020
Published: 17 June 2020
Accepted: 12 April 2020
Abstract Views: 1719
PDF: 927
HTML: 60
HTML: 60
Publisher's note
All claims expressed in this article are solely those of the authors and do not necessarily represent those of their affiliated organizations, or those of the publisher, the editors and the reviewers. Any product that may be evaluated in this article or claim that may be made by its manufacturer is not guaranteed or endorsed by the publisher.
All claims expressed in this article are solely those of the authors and do not necessarily represent those of their affiliated organizations, or those of the publisher, the editors and the reviewers. Any product that may be evaluated in this article or claim that may be made by its manufacturer is not guaranteed or endorsed by the publisher.
Similar Articles
- Thiago S. de Azevedo, Brian Patrick Bourke, Rafael Piovezan, Maria Anice M. Sallum, The influence of urban heat islands and socioeconomic factors on the spatial distribution of Aedes aegypti larval habitats , Geospatial Health: Vol. 13 No. 1 (2018)
- Manuel O. Espinosa, Francisco Polop, Camilo H. Rotela, Marcelo Abril, Carlos M. Scavuzzo, Spatial pattern evolution of Aedes aegypti breeding sites in an Argentinean city without a dengue vector control programme , Geospatial Health: Vol. 11 No. 3 (2016)
- Guillermo Albrieu-Llinás, Manuel O. Espinosa, Agustín Quaglia, Marcelo Abril, Carlos Marcelo Scavuzzo, Urban environmental clustering to assess the spatial dynamics of Aedes aegypti breeding sites , Geospatial Health: Vol. 13 No. 1 (2018)
- Joseph Leedale, Anne E. Jones, Cyril Caminade, Andrew P. Morse, A dynamic, climate-driven model of Rift Valley fever , Geospatial Health: Vol. 11 No. s1 (2016): HEALTHY FUTURES
- Ciro José Jardim de Figueiredo, Caroline Maria de Miranda Mota, Amanda Gadelha Ferreira Rosa, Arthur Pimentel Gomes de Souza, Simone Maria da Silva Lima, Vulnerability to COVID-19 in Pernambuco, Brazil: A geospatial evaluation supported by multiple-criteria decision aid methodology , Geospatial Health: Vol. 17 No. s1 (2022): Special issue on COVID-19
- Muhammad Aidil Roslan, Romano Ngui, Muhammad Fathi Marzuki, Indra Vythilingam, Aziz Shafie, Sabri Musa, Wan Yusoff Wan Sulaiman, Spatial dispersal of Aedes albopictus mosquitoes captured by the modified sticky ovitrap in Selangor, Malaysia , Geospatial Health: Vol. 17 No. 1 (2022)
- Camilo Rotela, Laura Lopez, María Frías Céspedes, Gabriela Barbas, Andrés Lighezzolo, Ximena Porcasi, Mario A. Lanfri, Carlos M. Scavuzzo, David E. Gorla, Analytical report of the 2016 dengue outbreak in Córdoba city, Argentina , Geospatial Health: Vol. 12 No. 2 (2017)
- João Batista Cavalcante Filho, Marco Aurélio de Oliveira Góes, Damião da Conceição Araújo, Marcus Valerius da Silva Peixoto, Marco Antônio Prado Nunes, Association of socioeconomic indicators with COVID-19 mortality in Brazil: a population-based ecological study , Geospatial Health: Vol. 18 No. 2 (2023)
- Amanda G. Carvalho, Carolina Lorraine H. Dias, David J. Blok, Eliane Ignotti, João Gabriel G. Luz, Intra-urban differences underlying leprosy spatial distribution in central Brazil: geospatial techniques as potential tools for surveillance , Geospatial Health: Vol. 18 No. 2 (2023)
- Valéria Miranda Avanzi, Udelysses Janete Veltrini Fonzar, Eraldo Schunk Silva, Jorge Juarez Vieira Teixeira, Dennis Armando Bertolini, Risk areas for hepatitis A, B and C in the municipality of Maringá, Paraná State, Brazil 2007-2010 , Geospatial Health: Vol. 13 No. 1 (2018)
You may also start an advanced similarity search for this article.