Spatiotemporal patterns of mortality associated with chronic non-communicable diseases and child malnutrition at the municipal level in Mexico
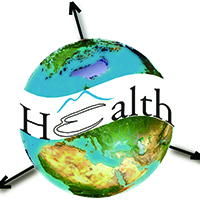
Accepted: 19 April 2022
Appendix: 86
HTML: 52
All claims expressed in this article are solely those of the authors and do not necessarily represent those of their affiliated organizations, or those of the publisher, the editors and the reviewers. Any product that may be evaluated in this article or claim that may be made by its manufacturer is not guaranteed or endorsed by the publisher.
Authors
Malnutrition is one of the main risk factors related to chronic non-communicable diseases and child undernourishment on a planetary scale. Mexico is one of the countries with the highest levels of malnutrition, but there is also an accelerated increase in overweight or obesity. This study explored the spatiotemporal behaviour of mortality associated with chronic non-communicable diseases such as type II diabetes mellitus, hypertension, ischemic heart disease and cerebrovascular disease. The analysis was carried out at the municipality level for the 2000-2020 period targeting two age groups: ≥60-year olds and 20-59-year olds. In addition, 0-4-year olds were investigated with respect to undernourishment. National databases were gathered and standardized for each disease and SaTScan spatiotemporal cluster analyses were performed. We found that mortality associated with most of the diseases evaluated has increased since 2016 except for mortality caused by child undernourishment, which showed a downward trend during the study period. To focus on active conglomerates of diseases is important as they currently represent a threat to public health. Our results contribute to the potential spatial prioritization of the allocation of resources and campaigns for prevention and treatment of chronic non-communicable diseases and child undernourishment. Generally, geographical studies are fundamental for the discovery of disease aetiology and they provide valuable and timely information to multiple stakeholders.
How to Cite

This work is licensed under a Creative Commons Attribution-NonCommercial 4.0 International License.
PAGEPress has chosen to apply the Creative Commons Attribution NonCommercial 4.0 International License (CC BY-NC 4.0) to all manuscripts to be published.