A spatio-temporal study of state-wide case-fatality risks during the first wave of the COVID-19 pandemic in Mexico
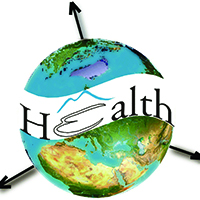
Accepted: 10 March 2022
Appendix: 147
HTML: 25
All claims expressed in this article are solely those of the authors and do not necessarily represent those of their affiliated organizations, or those of the publisher, the editors and the reviewers. Any product that may be evaluated in this article or claim that may be made by its manufacturer is not guaranteed or endorsed by the publisher.
Authors
spatio-temporal analysis of the first wave of the coronavirus (COVID-19) pandemic in Mexico (April to September 2020) was performed by state. Descriptive analyses through diagrams, mapping, animations and time series representations were carried out. Greater risks were observed at certain times in specific regions. Various trends and clusters were observed and analysed by fitting linear mixed models and time series clustering. The association of co-morbidities and other variables were studied by fitting a spatial panel data linear model (SPLM). On average, the greatest risks were observed in Baja California Norte, Chiapas and Sonora, while some other densely populated states, e.g., Mexico City, had lower values. The trends varied by state and a four-order polynomial, including fixed and random effects, was necessary to model them. The most common risk development was observed in states belonging to two clusters and consisted of an initial increase followed by a decrease. Some states presented cluster configurations with a retarded risk increase before the decrease, while the risk increased throughout the time of study in others. A cyclic behaviour with a second increasing trend was also observed in some states. The SPLM approach revealed a positive significant association with respect to case fatality risk between certain groups, such as males and individuals aged 50 years and more, and the prevalence of chronic kidney disease, cardiovascular disease, asthma and hypertension. The analysis may provide valuable insight into COVID-19 dynamics applicable in future outbreaks, as well as identify determinants signifying certain trends at the state level. The combination of spatial and temporal information may provide a better understanding of the fatalities due to COVID-19.
How to Cite

This work is licensed under a Creative Commons Attribution-NonCommercial 4.0 International License.
PAGEPress has chosen to apply the Creative Commons Attribution NonCommercial 4.0 International License (CC BY-NC 4.0) to all manuscripts to be published.