Spatiotemporal epidemiology of COVID-19 from an epidemic course perspective
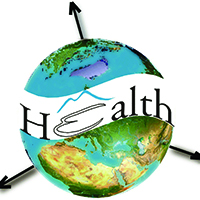
Submitted: 11 June 2021
Accepted: 16 August 2021
Published: 10 February 2022
Accepted: 16 August 2021
Abstract Views: 2536
PDF: 254
HTML: 19
HTML: 19
Publisher's note
All claims expressed in this article are solely those of the authors and do not necessarily represent those of their affiliated organizations, or those of the publisher, the editors and the reviewers. Any product that may be evaluated in this article or claim that may be made by its manufacturer is not guaranteed or endorsed by the publisher.
All claims expressed in this article are solely those of the authors and do not necessarily represent those of their affiliated organizations, or those of the publisher, the editors and the reviewers. Any product that may be evaluated in this article or claim that may be made by its manufacturer is not guaranteed or endorsed by the publisher.
Similar Articles
- Chunhui Liu, Xiaodi Su, Zhaoxuan Dong, Xingyu Liu, Chunxia Qiu, Understanding COVID-19: comparison of spatio-temporal analysis methods used to study epidemic spread patterns in the United States , Geospatial Health: Vol. 18 No. 1 (2023)
- Olga De Cos, Valentín Castillo-Salcines, David Cantarero-Prieto, A geographical information system model to define COVID-19 problem areas with an analysis in the socio-economic context at the regional scale in the North of Spain , Geospatial Health: Vol. 17 No. s1 (2022): Special issue on COVID-19
- Ei Sandar U, Wongsa Laohasiriwong, Kittipong Sornlorm, Spatial autocorrelation and heterogenicity of demographic and healthcare factors in the five waves of COVID-19 epidemic in Thailand , Geospatial Health: Vol. 18 No. 1 (2023)
- Amornrat Luenam, Nattapong Puttanapong , Spatial association between COVID-19 incidence rate and nighttime light index , Geospatial Health: Vol. 17 No. s1 (2022): Special issue on COVID-19
- Claire Bonzani, Peter Scull, Daisaku Yamamoto, A spatiotemporal analysis of the social determinants of health for COVID-19 , Geospatial Health: Vol. 18 No. 1 (2023)
- Ciro José Jardim de Figueiredo, Caroline Maria de Miranda Mota, Amanda Gadelha Ferreira Rosa, Arthur Pimentel Gomes de Souza, Simone Maria da Silva Lima, Vulnerability to COVID-19 in Pernambuco, Brazil: A geospatial evaluation supported by multiple-criteria decision aid methodology , Geospatial Health: Vol. 17 No. s1 (2022): Special issue on COVID-19
- Jerry Enoe , Michael Sutherland, Dexter Davis, Bheshem Ramlal, Charisse Griffith-Charles , Keston H. Bhola, Elsai Mati Asefa, A conceptional model integrating geographic information systems (GIS) and social media data for disease exposure assessment , Geospatial Health: Vol. 19 No. 1 (2024)
- Michał Paweł Michalak, Jack Cordes, Agnieszka Kulawik, Sławomir Sitek, Sławomir Pytel, Elżbieta Zuzańska-Żyśko, Radosław Wieczorek, Reducing bias in risk indices for COVID-19 , Geospatial Health: Vol. 17 No. s1 (2022): Special issue on COVID-19
- Nushrat Nazia, Spatial variations of COVID-19 risk by age in Toronto, Canada , Geospatial Health: Vol. 17 No. s1 (2022): Special issue on COVID-19
- Enner Alcântara, José Mantovani, Luiz Rotta, Edward Park, Thanan Rodrigues, Fernando Campos Carvalho, Carlos Roberto Souza Filho, Investigating spatiotemporal patterns of the COVID-19 in São Paulo State, Brazil , Geospatial Health: Vol. 15 No. 2 (2020)
You may also start an advanced similarity search for this article.