Using object-based image analysis to map commercial poultry operations from high resolution imagery to support animal health outbreaks and events
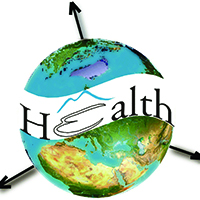
Accepted: 6 August 2020
HTML: 72
All claims expressed in this article are solely those of the authors and do not necessarily represent those of their affiliated organizations, or those of the publisher, the editors and the reviewers. Any product that may be evaluated in this article or claim that may be made by its manufacturer is not guaranteed or endorsed by the publisher.
Authors
Precise locations of commercial poultry operations are important to planning and response for animal health outbreaks and events. These data are not available nationally or uniformly in the United States. This project uses machine learning capabilities to identify and map commercial poultry operations from aerial imagery in seven south-eastern states in the United States. The output protocol uses an Object-Based Image Analysis (OBIA) approach, which identifies objects based on spectral signatures combined with spatial, contextual, and textural information. The protocol is a semi-automated and user-assisted process, meaning that the object identification routines require minimal user inputs or expertise. Using the protocol, we produced locations of likely commercial poultry operations in up to two counties in one workday, about two times faster than manual digitisation. The resulting datasets provide an estimate of the number and geographic distribution of commercial poultry operations to assist outbreak response by augmenting available knowledge in affected areas.
How to Cite

This work is licensed under a Creative Commons Attribution-NonCommercial 4.0 International License.
PAGEPress has chosen to apply the Creative Commons Attribution NonCommercial 4.0 International License (CC BY-NC 4.0) to all manuscripts to be published.