Modelling the domestic poultry population in the United States: A novel approach leveraging remote sensing and synthetic data methods
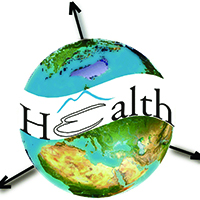
Accepted: 27 August 2020
HTML: 102
All claims expressed in this article are solely those of the authors and do not necessarily represent those of their affiliated organizations, or those of the publisher, the editors and the reviewers. Any product that may be evaluated in this article or claim that may be made by its manufacturer is not guaranteed or endorsed by the publisher.
Authors
Comprehensive and spatially accurate poultry population demographic data do not currently exist in the United States; however, these data are critically needed to adequately prepare for, and efficiently respond to and manage disease outbreaks. In response to absence of these data, this study developed a national-level poultry population dataset by using a novel combination of remote sensing and probabilistic modelling methodologies. The Farm Location and Agricultural Production Simulator (FLAPS) (Burdett et al., 2015) was used to provide baseline national-scale data depicting the simulated locations and populations of individual poultry operations. Remote sensing methods (identification using aerial imagery) were used to identify actual locations of buildings having the characteristic size and shape of commercial poultry barns. This approach was applied to 594 U.S. counties with > 100,000 birds in 34 states based on the 2012 U.S. Department of Agriculture (USDA), National Agricultural Statistics Service (NASS), Census of Agriculture (CoA). The two methods were integrated in a hybrid approach to develop an automated machine learning process to locate commercial poultry operations and predict the number and type of poultry for each operation across the coterminous United States. Validation illustrated that the hybrid model had higher locational accuracy and more realistic distribution and density patterns when compared to purely simulated data. The resulting national poultry population dataset has significant potential for application in animal disease spread modelling, surveillance, emergency planning and response, economics, and other fields, providing a versatile asset for further agricultural research.
How to Cite

This work is licensed under a Creative Commons Attribution-NonCommercial 4.0 International License.
PAGEPress has chosen to apply the Creative Commons Attribution NonCommercial 4.0 International License (CC BY-NC 4.0) to all manuscripts to be published.
Similar Articles
- Susan Maroney, MaryJane McCool-Eye, Andrew Fox, Christopher Burdett, Using object-based image analysis to map commercial poultry operations from high resolution imagery to support animal health outbreaks and events , Geospatial Health: Vol. 15 No. 2 (2020)
You may also start an advanced similarity search for this article.