Spatiality in small area estimation: A new structure with a simulation study
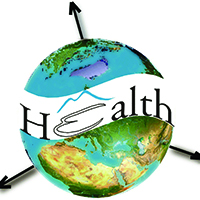
Accepted: 1 September 2020
Supplementary materials: 106
HTML: 11
All claims expressed in this article are solely those of the authors and do not necessarily represent those of their affiliated organizations, or those of the publisher, the editors and the reviewers. Any product that may be evaluated in this article or claim that may be made by its manufacturer is not guaranteed or endorsed by the publisher.
Authors
In numerous practical applications, data from neighbouring small areas present spatial correlation. More recently, an extension of the Fay-Herriot model through the Simultaneously Auto- Rregressive (SAR) process has been considered. The Conditional Auto-Regressive (CAR) structure is also a popular choice. The reasons of using these structures are theoretical properties, computational advantages and relative ease of interpretation. However, the assumption of the non-singularity of matrix (Im-ρW) is a problem. We introduce here a novel structure of the covariance matrix when approaching spatiality in small area estimation (SAE) comparing that with the commonly used SAR process. As an example, we present synthetic data on grape production with spatial correlation for 274 municipalities in the region of Tuscany as base data simulating data at each area and comparing the results. The SAR process had the smallest Root Average Mean Square Error (RAMSE) for all conditions. The RAMSE also generally decreased with increasing sample size. In addition, the RAMSE valuess did not show a specific behaviour but only spatially correlation coefficient changes led to a stronger decrease of RAMSE values than the SAR model when our new structure was applied. The new approach presented here is more flexible than the SAR process without severe increasing RAMSE values.
How to Cite

This work is licensed under a Creative Commons Attribution-NonCommercial 4.0 International License.
PAGEPress has chosen to apply the Creative Commons Attribution NonCommercial 4.0 International License (CC BY-NC 4.0) to all manuscripts to be published.