Spatial distribution and sociodemographic risk factors of malaria in Nigerian children less than 5 years old
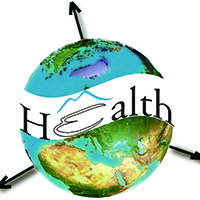
Accepted: 28 January 2020
HTML: 451
All claims expressed in this article are solely those of the authors and do not necessarily represent those of their affiliated organizations, or those of the publisher, the editors and the reviewers. Any product that may be evaluated in this article or claim that may be made by its manufacturer is not guaranteed or endorsed by the publisher.
Authors
Malaria remains a leading cause of morbidity and mortality among children in Nigeria less than 5 years old (under-5). This study utilized nationally representative secondary data extracted from the 2015 Nigeria Malaria Indicator Survey (NMIS) to investigate the spatial variability in malaria distribution in those under- 5 and to explore the influence of socioeconomic and demographic factors on malaria prevalence in this population group. To account for spatial correlation, a Spatially Generalized Linear Mixed Model (SGMM) was employed and predictive risk maps was developed using Kriging. Highly significant spatial variability in under-5 malaria distribution was observed (P<0.0001) with a higher likelihood of malaria prevalence in this group in the Northwest and North-east of the country. The number of malaria infections increased with age, children aged between 49-59 months were found to be at a higher risk (Odds Ratio=4.680, 95% CI=3.674 to 5.961 at P<0.0001). After accounting for spatial correlation, we observed a strong significant association between the non-availability or non-use of mosquito bed-nets, low household socioeconomic status, low level of mother's educational attainment, family size, anaemia prevalence, rural type of residence and under-5 malaria prevalence. Faced with a high rate of under-5 mortality due to malaria in Nigeria, targeted interventions (which requires the identification of the child's location) may reduce malaria prevalence, and we conclude that socioeconomic impediments need to be confronted to reduce the burden of childhood malaria infection.
How to Cite

This work is licensed under a Creative Commons Attribution-NonCommercial 4.0 International License.
PAGEPress has chosen to apply the Creative Commons Attribution NonCommercial 4.0 International License (CC BY-NC 4.0) to all manuscripts to be published.