Associating socio-economic factors with access to public healthcare facilities using geographically weighted regression in the city of Tshwane, South Africa
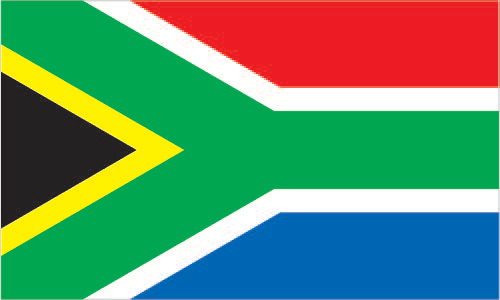
All claims expressed in this article are solely those of the authors and do not necessarily represent those of their affiliated organizations, or those of the publisher, the editors and the reviewers. Any product that may be evaluated in this article or claim that may be made by its manufacturer is not guaranteed or endorsed by the publisher.
Authors
Access to healthcare is influenced by various socio-economic factors such as income, population group, educational attainment and health insurance. This study used Geographically Weighted Regression (GWR) to investigate spatial variations in the association between socio-economic factors and access to public healthcare facilities in the City of Tshwane, South Africa based on data from the Gauteng City-Region Observatory Quality of Life Survey (2020/2021). Socio-economic predictors included population group, income, health insurance status and health satisfaction. The GWR model revealed that all socio-economic factors combined explained the variation in access to healthcare facilities (R²=0.77). Deviance residuals, ranging from -2.67 to 1.83, demonstrated a good model fit, indicating the robustness of the GWR model in predicting access to healthcare facilities. Black African, low-income and uninsured populations had each a relatively strong association with access to healthcare facilities (R²=0.65). Additionally, spatial patterns revealed that socio-economic relationships with access to health care facilities are not homogeneous, with significance of the relationships varying with space. This study highlights the need for a spatially nuanced approach to improving healthcare facilities access and emphasizes the need for targeted policy interventions that address local socio-environmental conditions.
How to Cite

This work is licensed under a Creative Commons Attribution-NonCommercial 4.0 International License.
PAGEPress has chosen to apply the Creative Commons Attribution NonCommercial 4.0 International License (CC BY-NC 4.0) to all manuscripts to be published.