Moran’s I and Geary’s C: investigation of the effects of spatial weight matrices for assessing the distribution of infectious diseases
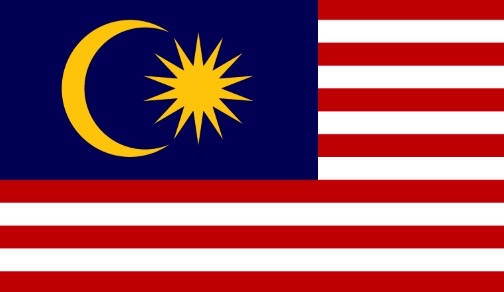
Published: 7 April 2025
Abstract Views: 209
PDF: 68
Supplementary materials: 7
Supplementary materials: 7
Publisher's note
All claims expressed in this article are solely those of the authors and do not necessarily represent those of their affiliated organizations, or those of the publisher, the editors and the reviewers. Any product that may be evaluated in this article or claim that may be made by its manufacturer is not guaranteed or endorsed by the publisher.
All claims expressed in this article are solely those of the authors and do not necessarily represent those of their affiliated organizations, or those of the publisher, the editors and the reviewers. Any product that may be evaluated in this article or claim that may be made by its manufacturer is not guaranteed or endorsed by the publisher.
Similar Articles
- Iuria Betco, Ana Isabel Ribeiro, David S. Vale, Luis Encalada-Abarca, Cláudia M. Viana, Jorge Rocha, Sentiment analysis using a lexicon-based approach in Lisbon, Portugal , Geospatial Health: Vol. 20 No. 1 (2025)
- Mai Liu, Yin Zhang, Impact of climate change on dengue fever: a bibliometric analysis , Geospatial Health: Vol. 20 No. 1 (2025)
- Beatriz Martínez-Lòpez, Tsviatko Alexandrov, Lina Mur, Fernando Sánchez-Vizcaíno, José M. Sánchez-Vizcaíno, Evaluation of the spatial patterns and risk factors, including backyard pigs, for classical swine fever occurrence in Bulgaria using a Bayesian model , Geospatial Health: Vol. 8 No. 2 (2014)
- Vitomir Djokić, Ivana Klun, Vincenzo Musella, Laura Rinaldi, Giuseppe Cringoli, Smaragda Sotiraki, Olgica Djurković-Djaković, Spatial epidemiology of Toxoplasma gondii infection in goats in Serbia , Geospatial Health: Vol. 8 No. 2 (2014)
- Ruy Brayner Oliveira Filho, Karla Campos Malta, Vania Lucia Assis Santana, Mabel Hanna Vance Harrop, Danilo Tancler Stipp, Daniel Friguglietti Brandespim, Rinaldo Aparecido Mota, José Júnior Wilton Pinheiro, Spatial characterization of Leptospira spp. infection in equids from the Brejo Paraibano micro-region in Brazil , Geospatial Health: Vol. 8 No. 2 (2014)
- Jia-Cheng Zhang, Wen-Dong Liu, Qi Liang, Jian-Li Hu, Jessie Norris, Ying Wu, Chang-Jun Bao, Fen-Yang Tang, Peng Huang, Yang Zhao, Rong-Bin Yu, Ming-Hao Zhou, Hong-Bing Shen, Feng Chen, Zhi-Hang Peng, Spatial distribution and risk factors of influenza in Jiangsu province, China, based on geographical information system , Geospatial Health: Vol. 8 No. 2 (2014)
- Naoko Nihei, Osamu Komagata, Kan-ichiro Mochizuki, Mutsuo Kobayashi, Geospatial analysis of invasion of the Asian tiger mosquito Aedes albopictus: competition with Aedes japonicus japonicus in its northern limit area in Japan , Geospatial Health: Vol. 8 No. 2 (2014)
- Rebekah S. Huber, Namkug Kim, Carl E. Renshaw, Perry F. Renshaw, Douglas G. Kondo, Relationship between altitude and lithium in groundwater in the United States of America: results of a 1992-2003 study , Geospatial Health: Vol. 9 No. 1 (2014)
- Luis E. Escobar, Andrés Lira-Noriega, Gonzalo Medina-Vogel, A. Townsend Peterson, Potential for spread of the white-nose fungus (Pseudogymnoascus destructans) in the Americas: use of Maxent and NicheA to assure strict model transference , Geospatial Health: Vol. 9 No. 1 (2014)
- Wendelin Moser, Helena Greter, Christian Schindler, Fiona Allan, Bongo N. R. Ngandolo, Daugla D. Moto, Jürg Utzinger, Jakob Zinsstag, The spatial and seasonal distribution of Bulinus truncatus, Bulinus forskalii and Biomphalaria pfeifferi, the intermediate host snails of schistosomiasis, in N'Djamena, Chad , Geospatial Health: Vol. 9 No. 1 (2014)
You may also start an advanced similarity search for this article.