Spatial pattern and heterogeneity of chronic respiratory diseases and relationship to socio-demographic factors in Thailand in the period 2016 to 2019
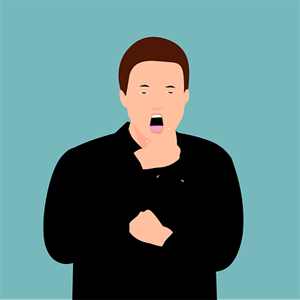
All claims expressed in this article are solely those of the authors and do not necessarily represent those of their affiliated organizations, or those of the publisher, the editors and the reviewers. Any product that may be evaluated in this article or claim that may be made by its manufacturer is not guaranteed or endorsed by the publisher.
Authors
Chronic respiratory diseases (CRDs) constitute 4% of the global disease burden and cause 4 million deaths annually. This cross-sectional study used QGIS and GeoDa to explore the spatial pattern and heterogeneity of CRDs morbidity and spatial autocorrelation between socio-demographic factors and CRDs in Thailand from 2016 to 2019. We found an annual, positive, spatial autocorrelation (Moran’s I >0.66, p<0.001) showing a strong clustered distribution. The local indicators of spatial association (LISA) identified hotspots mostly in the northern region, while coldspots were mostly seen in the central and north-eastern regions throughout the study period. Of the socio-demographic factors, the density of population, households, vehicles, factories and agricultural areas, correlated with the CRD morbidity rate, with statistically significant negative spatial autocorrelations and coldspots in the north-eastern and central areas (except for agricultural land) and two hotspots between farm household density and CRD in the southern region in 2019. This study identified vulnerable provinces with high risk of CRDs and can guide prioritization of resource allocation and provide target interventions for policy makers.
How to Cite

This work is licensed under a Creative Commons Attribution-NonCommercial 4.0 International License.
PAGEPress has chosen to apply the Creative Commons Attribution NonCommercial 4.0 International License (CC BY-NC 4.0) to all manuscripts to be published.