Province clustering based on the percentage of communicable disease using the BCBimax biclustering algorithm
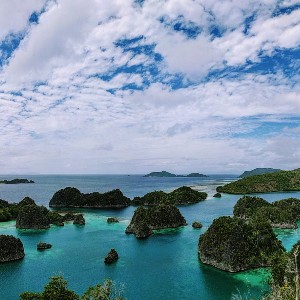
Accepted: 9 August 2023
HTML: 70
All claims expressed in this article are solely those of the authors and do not necessarily represent those of their affiliated organizations, or those of the publisher, the editors and the reviewers. Any product that may be evaluated in this article or claim that may be made by its manufacturer is not guaranteed or endorsed by the publisher.
Authors
Indonesia needs to lower its high infectious disease rate. This requires reliable data and following their temporal changes across provinces. We investigated the benefits of surveying the epidemiological situation with the imax biclustering algorithm using secondary data from a recent national scale survey of main infectious diseases from the National Basic Health Research (Riskesdas) covering 34 provinces in Indonesia. Hierarchical and k-means clustering can only handle one data source, but BCBimax biclustering can cluster rows and columns in a data matrix. Several experiments determined the best row and column threshold values, which is crucial for a useful result. The percentages of Indonesia’s seven most common infectious diseases (ARI, pneumonia, diarrhoea, tuberculosis (TB), hepatitis, malaria, and filariasis) were ordered by province to form groups without considering proximity because clusters are usually far apart. ARI, pneumonia, and diarrhoea were divided into toddler and adult infections, making 10 target diseases instead of seven. The set of biclusters formed based on the presence and level of these diseases included 7 diseases with moderate to high disease levels, 5 diseases (formed by 2 clusters), 3 diseases, 2 diseases, and a final order that only included adult diarrhoea. In 6 of 8 clusters, diarrhea was the most prevalent infectious disease in Indonesia, making its eradication a priority. Direct person-to-person infections like ARI, pneumonia, TB, and diarrhoea were found in 4-6 of 8 clusters. These diseases are more common and spread faster than vector-borne diseases like malaria and filariasis, making them more important.
How to Cite

This work is licensed under a Creative Commons Attribution-NonCommercial 4.0 International License.
PAGEPress has chosen to apply the Creative Commons Attribution NonCommercial 4.0 International License (CC BY-NC 4.0) to all manuscripts to be published.