Understanding the spatial non-stationarity in the relationships between malaria incidence and environmental risk factors using Geographically Weighted Random Forest: A case study in Rwanda
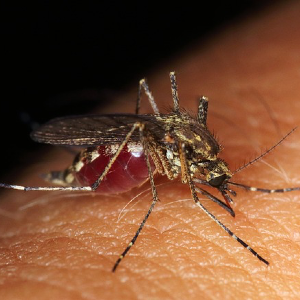
Accepted: 28 April 2023
HTML: 61
All claims expressed in this article are solely those of the authors and do not necessarily represent those of their affiliated organizations, or those of the publisher, the editors and the reviewers. Any product that may be evaluated in this article or claim that may be made by its manufacturer is not guaranteed or endorsed by the publisher.
Authors
As found in the health studies literature, the levels of climate association between epidemiological diseases have been found to vary across regions. Therefore, it seems reasonable to allow for the possibility that relationships might vary spatially within regions. We implemented the geographically weighted random forest (GWRF) machine learning method to analyze ecological disease patterns caused by spatially non-stationary processes using a malaria incidence dataset for Rwanda. We first compared the geographically weighted regression (WGR), the global random forest (GRF), and the geographically weighted random forest (GWRF) to examine the spatial non-stationarity in the non-linear relationships between malaria incidence and their risk factors. We used the Gaussian areal kriging model to disaggregate the malaria incidence at the local administrative cell level to understand the relationships at a fine scale since the model goodness of fit was not satisfactory to explain malaria incidence due to the limited number of sample values. Our results show that in terms of the coefficients of determination and prediction accuracy, the geographical random forest model performs better than the GWR and the global random forest model. The coefficients of determination of the geographically weighted regression (R2), the global RF (R2), and the GWRF (R2) were 4.74, 0.76, and 0.79, respectively. The GWRF algorithm achieves the best result and reveals that risk factors (rainfall, land surface temperature, elevation, and air temperature) have a strong non-linear relationship with the spatial distribution of malaria incidence rates, which could have implications for supporting local initiatives for malaria elimination in Rwanda.
How to Cite

This work is licensed under a Creative Commons Attribution-NonCommercial 4.0 International License.
PAGEPress has chosen to apply the Creative Commons Attribution NonCommercial 4.0 International License (CC BY-NC 4.0) to all manuscripts to be published.