Impacts of sample ratio and size on the performance of random forest model to predict the potential distribution of snail habitats
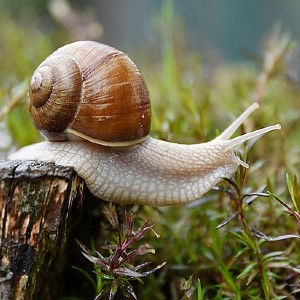
Accepted: 8 February 2023
Supplementary Materials: 56
HTML: 29
All claims expressed in this article are solely those of the authors and do not necessarily represent those of their affiliated organizations, or those of the publisher, the editors and the reviewers. Any product that may be evaluated in this article or claim that may be made by its manufacturer is not guaranteed or endorsed by the publisher.
Authors
Few studies have considered the impacts of sample size and sample ratio of presence and absence points on the results of random forest (RF) testing. We applied this technique for the prediction of the spatial distribution of snail habitats based on a total of 15,000 sample points (5,000 presence samples and 10,000 control points). RF models were built using seven different sample ratios (1:1, 1:2, 1:3, 1:4, 2:1, 3:1, and 4:1) and the optimal ratio was identified via the Area Under the Curve (AUC) statistic. The impact of sample size was compared by RF models under the optimal ratio and the optimal sample size. When the sample size was small, the sampling ratios of 1:1, 1:2 and 1:3 were significantly better than the sample ratios of 4:1 and 3:1 at all four levels of sample sizes (p<0.01) and there was no significant difference among the ratios of 1:1, 1:2 and 1:3 (p>0.05). The sample ratio of 1:2 appeared to be optimal for a relatively large sample size with the lowest quartile deviation. In addition, increasing the sample size produced a higher AUC and a smaller slope and the most suitable sample size found in this study was 2400 (AUC=0.96). This study provides a feasible idea to select an appropriate sample size and sample ratio for ecological niche modelling (ENM) and also provides a scientific basis for the selection of samples to accurately identify and predict snail habitat distributions.
How to Cite

This work is licensed under a Creative Commons Attribution-NonCommercial 4.0 International License.
PAGEPress has chosen to apply the Creative Commons Attribution NonCommercial 4.0 International License (CC BY-NC 4.0) to all manuscripts to be published.