Spatial autocorrelation and stratified heterogeneity in the evaluation of breast cancer risk inequity and socioeconomic factors analysis in China: Evidence from Nanchang, Jiangxi Province
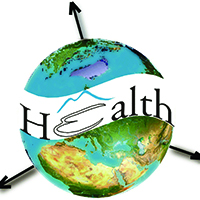
All claims expressed in this article are solely those of the authors and do not necessarily represent those of their affiliated organizations, or those of the publisher, the editors and the reviewers. Any product that may be evaluated in this article or claim that may be made by its manufacturer is not guaranteed or endorsed by the publisher.
Authors
Study of socioeconomic factors can play an important role in the spatial distribution of breast cancer by leading to a better understanding of its spatial pattern and assist breast cancer screening and early diagnosis. Taking Nanchang, a major city in central China, as an example, spatial autocorrelation and stratified heterogeneity were applied using a 10 10 km grid division to analyse breast cancer risk and socioeconomic factors. The research results showed that the median incidence rate of female breast cancer in Nanchang from 2016 to 2018 was 6.6/100,000 with a standard deviation of 12.3/100,000. Areas with higher incidence rates were mainly located in the central urban area and the major county towns. Spatial regression analysis showed that there was a statistically significant correlation between the spatial patterns of breast cancer incidence on the one hand, and on the other socioeconomic factors, such as total gross domestic product (GDP), per capita GDP and density of places of social and economic activities, i.e. points of interest. In addition, the normalized difference vegetation index also played a part in this respect. This research could serve as a reference for regional public health policy formulation and breast cancer screening.
How to Cite

This work is licensed under a Creative Commons Attribution-NonCommercial 4.0 International License.
PAGEPress has chosen to apply the Creative Commons Attribution NonCommercial 4.0 International License (CC BY-NC 4.0) to all manuscripts to be published.