Post-pandemic COVID-19 estimated and forecasted hotspots in the Association of Southeast Asian Nations (ASEAN) countries in connection to vaccination rate
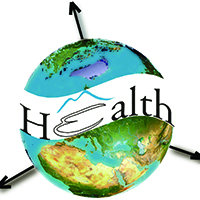
Submitted: 9 January 2022
Accepted: 8 March 2022
Published: 22 March 2022
Accepted: 8 March 2022
Abstract Views: 1096
PDF: 294
HTML: 31
HTML: 31
Publisher's note
All claims expressed in this article are solely those of the authors and do not necessarily represent those of their affiliated organizations, or those of the publisher, the editors and the reviewers. Any product that may be evaluated in this article or claim that may be made by its manufacturer is not guaranteed or endorsed by the publisher.
All claims expressed in this article are solely those of the authors and do not necessarily represent those of their affiliated organizations, or those of the publisher, the editors and the reviewers. Any product that may be evaluated in this article or claim that may be made by its manufacturer is not guaranteed or endorsed by the publisher.
Similar Articles
- Yunho Yeom, Jaehun Choi, The spatiotemporal dynamics and structural covariates of homicide in the Republic of Korea, 2008-2017: A dynamic spatial panel data approach , Geospatial Health: Vol. 17 No. 1 (2022)
- Zhao Rong Huang, Miao Ge, Xin Rui Pang, Pu Song, Congxia Wang, The spatial distribution of interleukin-4 (IL-4) reference values in China based on a back propagation (BP) neural network , Geospatial Health: Vol. 18 No. 2 (2023)
- Aline Beatriz dos Santos Silva, Ana Catarina de Melo Araújo, Amanda Priscila de Santana Cabral Silva, Mirella Bezerra Rodrigues Vilela, Cristine Vieira do Bonfim, Spatial patterns of the total mortality over the first 24 hours of life and that due to preventable causes , Geospatial Health: Vol. 17 No. 1 (2022)
- Diana Gomez-Barroso, Emilia Velasco, Carmen Varela, Inmaculada Leon, Rosa Cano, Spread of Ebola virus disease based on the density of roads in West Africa , Geospatial Health: Vol. 12 No. 2 (2017)
- Roberto Condoleo, Vincenzo Musella, Maria Paola Maurelli, Antonio Bosco, Giuseppe Cringoli, Laura Rinaldi, Mapping, cluster detection and evaluation of risk factors of ovine toxoplasmosis in Southern Italy , Geospatial Health: Vol. 11 No. 2 (2016)
- Gianluca Boo, Stefan Leyk, Sara Irina Fabrikant, Andreas Pospischil, Ramona Graf, Assessing effects of structural zeros on models of canine cancer incidence: a case study of the Swiss Canine Cancer Registry , Geospatial Health: Vol. 12 No. 1 (2017)
- Kubra Boz, Hayri Hakan Denli, Spatial electromagnetic field intensity modelling of global system for mobile communication base stations in the Istanbul Technical University Ayazaga campus area , Geospatial Health: Vol. 13 No. 1 (2018)
- Seong-Yong Park, Jin-Mi Kwak, Eun-Won Seo, Kwang-Soo Lee, Spatial analysis of the regional variation of hypertensive disease mortality and its socio-economic correlates in South Korea , Geospatial Health: Vol. 11 No. 2 (2016)
- Stephen Thielke, Christopher G. Slatore, William A. Banks, Quantifying altitude of human habitation in studies of human health using geographical name server data , Geospatial Health: Vol. 11 No. 3 (2016)
- Sue C. Grady, April N. Frake, Qiong Zhang, Matlhogonolo Bene, Demetrice R. Jordan, Joshua Vertalka, Thania C. Dossantos, Ameen Kadhim, Judith Namanya, Lisa-Marie Pierre, Yi Fan, Peiling Zhou, Fatoumata B. Barry, Libbey Kutch, Neonatal mortality in East Africa and West Africa: a geographic analysis of district-level demographic and health survey data , Geospatial Health: Vol. 12 No. 1 (2017)
<< < 12 13 14 15 16 17 18 19 20 21 > >>
You may also start an advanced similarity search for this article.