A spatiotemporal analysis of COVID-19 transmission in Jakarta, Indonesia for pandemic decision support
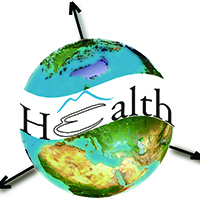
Accepted: 8 November 2021
HTML: 68
All claims expressed in this article are solely those of the authors and do not necessarily represent those of their affiliated organizations, or those of the publisher, the editors and the reviewers. Any product that may be evaluated in this article or claim that may be made by its manufacturer is not guaranteed or endorsed by the publisher.
Authors
With 25% confirmed cases of the country's total number of coronavirus disease 2019 (COVID-19) on 31 January 2021, Jakarta has the highest confirmed cases of in Indonesia. The city holds a significant role as the centre of government and national economic activity for which pandemic have had a huge impact. Spatiotemporal analysis was employed to identify the current condition of disease transmission and to provide comprehensive information on the COVID-19 outbreak in Jakarta. We applied space-time analysis to visualise the pattern of COVID-19 hotspots in each time series. We also mapped area capacity of the referral hospitals covering the entire area of Jakarta to understand the hospital service range. This research was conducted in 4 stages: i) disease mapping; ii) spatial autocorrelation analysis; iii) space-time pattern analysis; and iv) areal capacity mapping. The analysis resulted in 144 sub-districts categorised as high vulnerability. Autocorrelation studies by Moran's I identified cluster patterns and the emerging hotspot results indicated successful interventions as the number of hotspots fell in the first period of social restrictions. The results presented should be beneficial for policy makers.
How to Cite

This work is licensed under a Creative Commons Attribution-NonCommercial 4.0 International License.
PAGEPress has chosen to apply the Creative Commons Attribution NonCommercial 4.0 International License (CC BY-NC 4.0) to all manuscripts to be published.
Similar Articles
- Amanda G. Carvalho, Carolina Lorraine H. Dias, David J. Blok, Eliane Ignotti, João Gabriel G. Luz, Intra-urban differences underlying leprosy spatial distribution in central Brazil: geospatial techniques as potential tools for surveillance , Geospatial Health: Vol. 18 No. 2 (2023)
- Farrah Fahdhienie, Frans Yosep Sitepu, Spatio-temporal analysis of tuberculosis incidence in North Aceh District, Indonesia 2019-2021 , Geospatial Health: Vol. 17 No. 2 (2022)
You may also start an advanced similarity search for this article.