Vulnerability to COVID-19 in Pernambuco, Brazil: A geospatial evaluation supported by multiple-criteria decision aid methodology
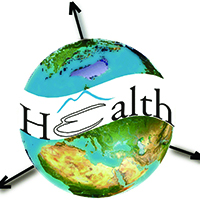
Accepted: 8 July 2021
HTML: 39
All claims expressed in this article are solely those of the authors and do not necessarily represent those of their affiliated organizations, or those of the publisher, the editors and the reviewers. Any product that may be evaluated in this article or claim that may be made by its manufacturer is not guaranteed or endorsed by the publisher.
Authors
The paper presents an innovative application to identify areas vulnerable to coronavirus disease 2019 (COVID-19) considering a combination of spatial analysis and a multi-criteria learning approach. We applied this methodology in the state of Pernambuco, Brazil identifying vulnerable areas by considering a set of determinants and risk factors for COVID-19, including demographic, economic and spatial characteristics and the number of human COVID-19 infections. Examining possible patterns over a set number of days taking the number of cases recorded, we arrived at a set of compatible decision rules to explain the relation between risk factors and COVID-19 cases. The results reveal why certain municipalities are critically vulnerable to COVID-19 highlighting locations for which knowledge can be gained about environmental factors.
How to Cite

This work is licensed under a Creative Commons Attribution-NonCommercial 4.0 International License.
PAGEPress has chosen to apply the Creative Commons Attribution NonCommercial 4.0 International License (CC BY-NC 4.0) to all manuscripts to be published.
-
Olga De Cos, Valentín Castillo-Salcines, David Cantarero-PrietoGeospatial Health : 2022
-
Edward Abel, Sajid SirajApplied Soft Computing : 2024
-
E. Mondino, A. Scolobig, G. Di Baldassarre, M. StoffelInternational Journal of Disaster Risk Reduction : 2023